Spotify Playlist Algorithm Deep Dive
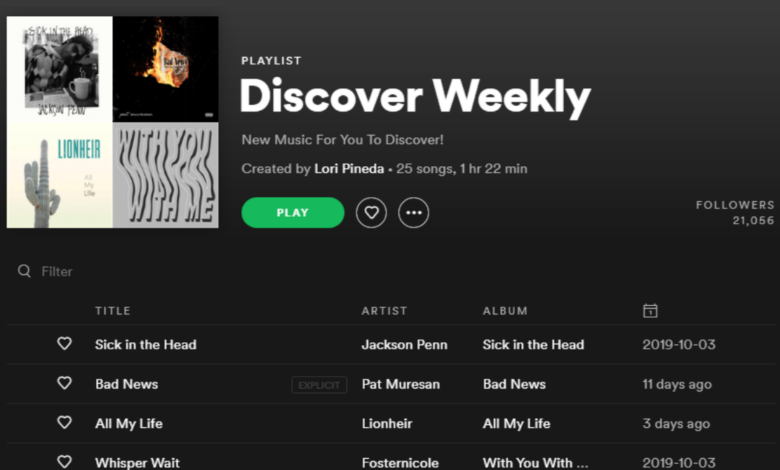
Unveiling the lista reproduccion spotify algoritmo, this exploration delves into the intricate workings of Spotify’s playlist algorithms. How do these algorithms curate the perfect listening experience, influencing our musical tastes and shaping the very fabric of our daily audio journeys?
From collaborative playlists that reflect the tastes of your friends to personalized recommendations that anticipate your next favorite song, the algorithms are a powerful force in the streaming world. We’ll dissect the different types of algorithms, analyze their impact on user behavior, and investigate the role they play in the music industry. This detailed analysis will help you understand how Spotify’s playlist algorithms shape your musical world.
Spotify Playlists & Algorithms: Lista Reproduccion Spotify Algoritmo
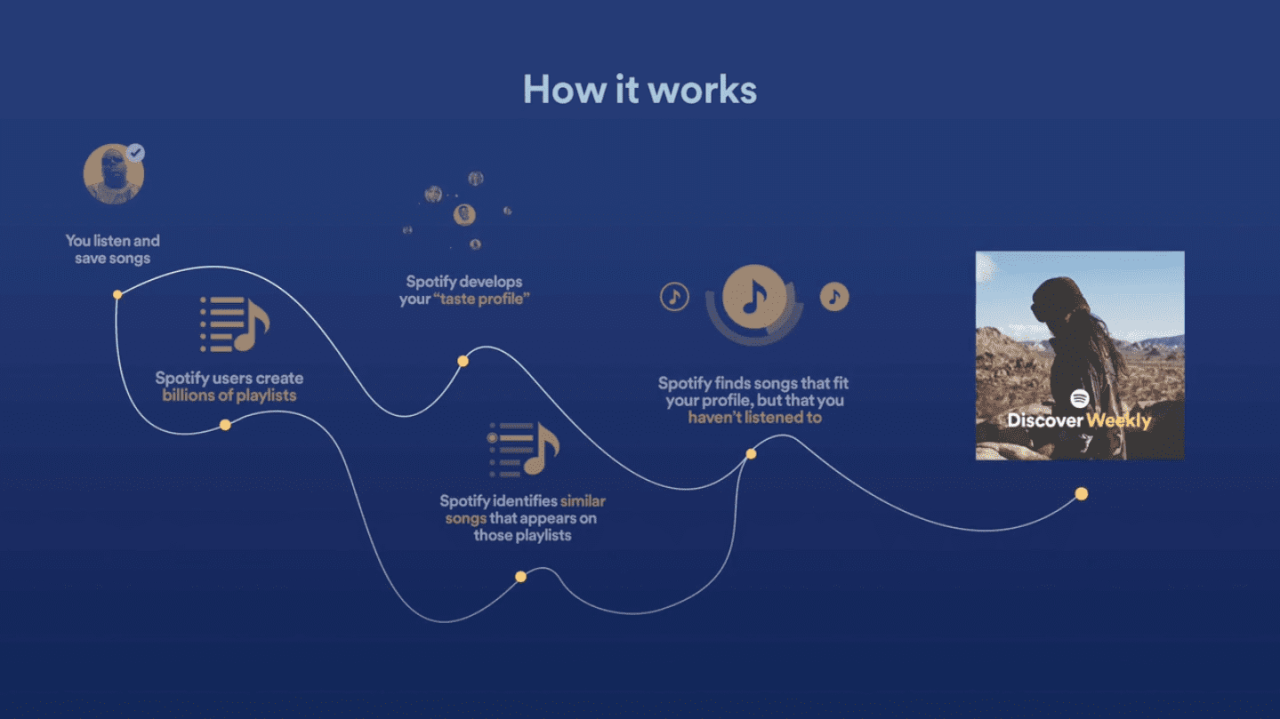
Spotify’s vast library of music is meticulously organized and presented through its powerful playlist system. Behind the scenes, sophisticated algorithms work tirelessly to curate these playlists, tailoring musical experiences to individual tastes and preferences. This intricate process involves a combination of collaborative and personalized approaches, resulting in a diverse range of playlists for every mood and occasion. Understanding these algorithms is key to appreciating the personalized music recommendations that shape our listening habits.Spotify’s algorithms are not static entities; they constantly evolve, learning from user interactions and incorporating new data to refine their recommendations.
This dynamic nature allows Spotify to adapt to shifting musical trends and individual preferences, providing users with fresh and relevant listening experiences. These dynamic adjustments are fundamental to maintaining the platform’s relevance and user engagement.
Playlist Algorithm Types
Spotify utilizes a multifaceted approach to playlist creation, blending collaborative and personalized algorithms. This approach allows users to discover new music while maintaining a personalized listening experience. Collaborative algorithms leverage the listening habits of a broader community, while personalized algorithms cater to individual preferences. This combination leads to a diverse and engaging musical landscape on the platform.
Collaborative Algorithms
These algorithms identify commonalities in listening habits across users. By observing which songs and artists are frequently enjoyed together, Spotify can generate playlists that reflect shared musical tastes. This approach is particularly useful for discovering new music that aligns with broader trends or genres. For instance, if a significant portion of users listening to jazz also enjoy smooth jazz, Spotify might create a playlist specifically for smooth jazz enthusiasts, based on this observation.
These playlists often showcase popular or emerging musical trends, broadening a user’s exposure to music they might not have encountered otherwise.
Personalized Algorithms
These algorithms meticulously analyze individual user listening patterns to curate playlists that are uniquely tailored to their preferences. Spotify meticulously tracks the songs, artists, and genres that a user frequently listens to, allowing it to predict with remarkable accuracy what other music they might enjoy. This personalized approach results in highly targeted recommendations, ensuring that users are consistently exposed to music that resonates with their individual tastes.
For example, a user who frequently listens to indie rock and alternative artists will likely receive recommendations for similar artists and genres. This personalization is a key factor in Spotify’s success, fostering a strong connection between the platform and its users.
Bias in Algorithms
Spotify’s algorithms, despite their sophistication, are not immune to potential biases. These biases can stem from the data they are trained on, which may not fully represent the diversity of musical tastes across different demographics. For example, if a particular music genre is disproportionately represented in the platform’s dataset, the algorithm may favor that genre in its recommendations.
My Spotify playlists are always a fascinating study in algorithms. I’ve been wondering how the “lista reproduccion spotify algoritmo” works lately, especially given the recent New Hampshire Democratic primary results. Those results, available at results new hampshire democratic primary , show some interesting trends, and I’m guessing the Spotify algorithm is taking those into account, tailoring my recommendations based on the current political climate.
So, I’m back to wondering about the specifics of the “lista reproduccion spotify algoritmo” again.
This can lead to a lack of diversity in the recommendations and limit the exposure of underrepresented genres.
Comparison of Algorithm Types
Algorithm Type | Description | User Impact | Potential Biases |
---|---|---|---|
Collaborative | Identifies common listening patterns among users to create playlists reflecting shared tastes. | Discovers new music aligned with broader trends; potential exposure to diverse genres. | May favor popular or over-represented genres; may not fully reflect individual tastes. |
Personalized | Analyzes individual user listening history to create tailored playlists based on preferences. | Highly targeted recommendations; consistent exposure to music that resonates with individual tastes. | May perpetuate existing preferences, potentially limiting exposure to new genres; can be influenced by the initial dataset’s representation. |
Impact on User Behavior
Spotify’s playlist algorithms have a profound impact on user behavior, shaping not only their music choices but also their overall listening experience and how they discover new artists and genres. These algorithms, constantly learning and adapting to user preferences, act as a personalized curator, guiding listeners through a vast musical landscape. The influence extends beyond passive listening, impacting how users actively engage with music and create their own playlists.Spotify’s algorithms are designed to anticipate user preferences and present music tailored to their existing tastes.
This personalized approach can lead to both positive and negative consequences, shaping listening habits and potentially limiting exposure to diverse musical styles. Understanding how these algorithms function is key to comprehending their impact on the listening experience.
Music Discovery
Playlist algorithms are instrumental in introducing users to new music. By analyzing listening history, and preferences, the algorithm identifies potential matches based on existing favorites. This targeted approach allows users to explore related artists and genres, gradually broadening their musical horizons. The process of discovery is often a serendipitous one, where users encounter new artists and styles they might not have discovered independently.
For example, a user initially enjoying pop music might be introduced to alternative rock through a playlist recommendation, broadening their musical palette.
Playlist Creation
Spotify’s algorithms empower users to create playlists that reflect their diverse moods and interests. By analyzing listening patterns and preferences, the algorithm can suggest songs and artists that fit the theme or mood of an existing playlist. This assistance makes playlist creation easier and more efficient. Furthermore, the algorithm allows users to categorize and structure their music collections based on personalized criteria.
The availability of pre-made playlists and the ability to tailor existing playlists further simplifies the process.
Listening Habits
The algorithms influence listening habits in a significant way. Users often rely on curated playlists for daily listening, establishing a consistent flow of music that aligns with their moods or activities. The algorithm’s ability to anticipate and cater to those preferences leads to a more enjoyable and engaging listening experience. This predictability can, however, potentially limit exploration outside of the algorithm’s suggestions, creating a ‘filter bubble’ effect.
Potential Limitations
While playlist algorithms offer numerous benefits, there are inherent limitations to consider. The algorithm’s focus on existing preferences can lead to a “filter bubble,” where users are primarily exposed to music similar to what they already listen to, potentially hindering exposure to diverse musical styles and artists. This can be particularly concerning for users with limited musical experience, who may not have developed a broad enough range of preferences to trigger varied recommendations.
Ever wondered how Spotify’s algorithm crafts those perfect playlists? It’s fascinating, right? Similarly, understanding the complexities of political processes like the Nevada caucus primary is also quite interesting. A good explainer, like the Nevada caucus primary explainer , can help you grasp the nuances of the process. Ultimately, both the Spotify algorithm and the political process are designed to match interests and preferences, leading to personalized experiences, whether it’s music or political choices.
The algorithmic curation may also overlook niche genres or emerging artists, limiting the breadth of musical discovery.
Table of User Behaviors Influenced by Spotify’s Playlist Algorithms
User Behavior | Algorithm Influence | Example |
---|---|---|
Music Discovery | Algorithms identify potential matches based on existing listening history, leading to the discovery of new artists and genres. | A user initially enjoying pop music is introduced to alternative rock through a playlist recommendation. |
Playlist Creation | Algorithms suggest songs and artists that fit the theme or mood of an existing playlist, simplifying the process and enabling users to create playlists more effectively. | A user creates a “workout” playlist, and the algorithm suggests upbeat tracks that match the mood. |
Listening Habits | Algorithms provide a consistent flow of music tailored to users’ moods or activities, leading to a more enjoyable listening experience. | A user listening to a “relaxing” playlist during their commute experiences a sense of calm. |
Playlist Creation & Customization
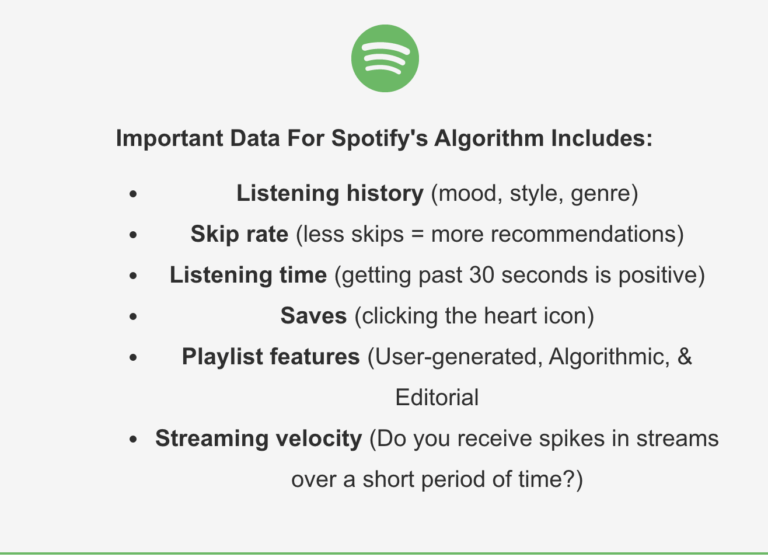
Spotify’s playlist feature empowers users to curate personalized auditory experiences. This goes beyond simply assembling songs; it’s a dynamic process that allows for tailoring and refinement, significantly impacting the user experience and the algorithm’s learning process. Understanding the mechanisms of playlist creation and customization is crucial for harnessing the full potential of the platform and shaping personalized recommendations.
Creating Playlists
The process of creating a playlist on Spotify is straightforward and intuitive. Users initiate the process by selecting the “Create Playlist” option, typically found within the main menu or dedicated playlist section. This opens a window where users can input the playlist’s name and description. Choosing a suitable name, which can be as specific or broad as desired, is a fundamental step.
A brief description aids in the categorization and recall of the playlist later on. From this point, users can either directly add songs from their library or from the extensive Spotify catalog.
Customizing Playlists
Beyond basic song addition, Spotify provides numerous options for refining and shaping a playlist. Users can refine their playlist’s genre, mood, or tempo. This allows for more targeted and focused listening experiences. Further customization options encompass the ability to set a specific order for songs within a playlist, enabling a curated listening experience that aligns with the user’s preferences.
Features like “Smart Shuffle” allow for randomized listening sessions while still retaining the user’s specified playlist content. Users can also add collaborative elements by sharing playlists with friends, fostering communal music discovery.
Influencing Algorithm Recommendations
Users play a significant role in shaping Spotify’s algorithm recommendations through playlist creation. By carefully selecting songs and categorizing playlists, users provide valuable data points for the algorithm to analyze. The algorithm learns from the user’s choices, preferences, and listening patterns. The more specific and consistent a user’s playlist selections are, the more refined the algorithm’s recommendations become.
Users also influence algorithm recommendations by utilizing the platform’s “save” and “like” features, as these actions communicate explicit interest to the system.
User Input and Playlist Generation
User input significantly impacts the playlist generation process. The more songs a user adds to a playlist, the more context the algorithm has to understand the user’s taste. Users can influence playlist generation by adding specific genres or artists to their playlists. For example, if a user consistently adds songs from indie artists to their “Chill Vibes” playlist, the algorithm is likely to recommend similar indie artists and songs in the future.
The frequency and consistency of this input are critical to the accuracy and relevance of algorithm-generated recommendations.
Playlist Creation Flow Chart
Step | Action |
---|---|
1 | Open Spotify and navigate to the playlist creation section. |
2 | Input a name and description for the playlist. |
3 | Select songs to add from the library or the Spotify catalog. |
4 | (Optional) Customize the playlist using options like genre, mood, or tempo. |
5 | (Optional) Set the song order. |
6 | (Optional) Share the playlist with friends. |
7 | Save the playlist. |
Algorithm’s Role in Music Industry
Spotify’s algorithms have revolutionized the music industry, fundamentally altering how artists gain exposure, how users discover music, and how the entire ecosystem functions. The platform’s sophisticated algorithms aren’t just about matching songs to listeners; they’re a powerful force shaping music trends and impacting the financial health of artists and labels.The algorithms play a crucial role in directing music towards the right audience.
This precise targeting, in turn, affects not only how songs perform but also how entire genres or artists gain traction. This dynamic interplay between algorithms, user preferences, and artist promotion is a core element of Spotify’s success and the modern music landscape.
Impact on Artists’ Exposure and Popularity
Spotify’s algorithms significantly impact an artist’s visibility and popularity. By analyzing user listening habits and preferences, the platform can recommend artists and songs to new audiences. This targeted exposure can lead to increased streams, downloads, and ultimately, greater financial success. Artists with a strong presence in curated playlists or those whose music resonates with the algorithm’s recommendations see their popularity grow exponentially.
For example, a lesser-known artist featured prominently in a “New Music Friday” playlist can rapidly gain a substantial following. Conversely, artists whose music doesn’t align with current trends or user preferences may struggle to gain traction.
Role of Playlists in Shaping Music Trends
Spotify’s playlists, heavily influenced by the algorithms, act as powerful trendsetters. Curated playlists often introduce new artists and songs to a broad audience, instantly raising their profile. The algorithms analyze user listening data and create playlists that reflect current popular trends, influencing what’s considered “in” and what’s likely to become popular. For instance, a playlist dedicated to “Chill vibes” might propel a specific genre or a collection of artists into prominence, effectively shaping the musical landscape.
Algorithm’s Impact on Music Discovery for Artists
The algorithm also plays a crucial role in how artists discover other music. Artists using Spotify can analyze the platform’s recommendations to identify emerging trends and gain insights into what resonates with audiences. This allows artists to adjust their music, marketing strategies, and overall artistic direction to better meet listener expectations. This data-driven approach empowers artists to adapt their work and improve their music to stay relevant.
Impact on Different Stakeholders
Stakeholder | Positive Impact | Negative Impact |
---|---|---|
Artists | Increased exposure and potential for wider audience reach. Ability to identify emerging trends. Potential for increased financial success through streams and licensing deals. | Dependence on the algorithm for visibility, potential for skewed metrics if the algorithm isn’t aligned with their style, struggle to gain traction with a unique style or less-commercial approach. |
Labels | Improved visibility for their artists, access to data on listener preferences, potential for new revenue streams. | Increased pressure to produce music that aligns with the algorithm’s favored trends, potential for underrepresentation of less-commercialized genres. |
Users | Personalized music recommendations, access to a vast library of music, discovery of new artists and genres. | Potential for “filter bubbles” where users only hear music similar to what they already listen to, algorithm bias may lead to limited exposure to diverse musical styles. |
Future Trends & Innovations
Spotify’s playlist algorithms are constantly evolving, driven by advancements in technology and the ever-changing tastes of its users. The future of these algorithms promises even more personalized and insightful music experiences, going beyond simple genre matching to truly understand and anticipate user preferences. This evolution hinges on integrating emerging technologies and leveraging the power of artificial intelligence.
Potential Developments in Playlist Algorithms
Spotify’s current algorithms excel at matching users with music based on genre, artist, and mood. Future iterations will likely incorporate more sophisticated techniques, including machine learning models that can analyze user listening habits across multiple platforms and devices. This approach will go beyond simple song-level analysis to consider the context of listening, such as time of day, location, and social interactions.
These advancements will result in more nuanced and relevant recommendations, anticipating user needs and desires more effectively.
Ever wondered how Spotify’s algorithm crafts those perfect playlists? It’s fascinating how it anticipates your musical tastes. Choosing a baby’s name, though, is a whole different ballgame. It’s often influenced by family heritage and traditions, like deciding on a last name for the little one. For a deeper dive into the world of naming and the factors that go into choosing a child’s last name, check out this article on apellido bebe madre padre.
Ultimately, whether it’s Spotify’s playlist genius or naming a baby, there’s a fascinating algorithm at play. I’m definitely going to have to experiment with the Spotify algorithm again now I know this!
Emerging Technologies Influencing Playlist Creation, Lista reproduccion spotify algoritmo
Emerging technologies like natural language processing (NLP) and sentiment analysis are poised to significantly impact playlist creation. NLP allows the algorithm to understand the nuances of user feedback and reviews, including lyrics, artist statements, and social media discussions. Sentiment analysis, in turn, will allow the algorithm to gauge user emotions associated with specific songs or artists, enabling playlists tailored to specific emotional states.
Ever wondered how Spotify’s algorithm crafts those perfect playlists? It’s fascinating how the lista reproduccion spotify algorithm works, constantly learning and adapting to your listening habits. While that’s happening, it’s also worth noting the influence of corporate power, like in the case of the Koch Chevron deference supreme court decisions, which often shape policy impacting everything from environmental regulations to energy production.
Ultimately, understanding these complex relationships gives us a deeper appreciation for the ever-evolving world of music streaming and the wider political landscape, all tied to the intricate lista reproduccion spotify algorithm. koch chevron deference supreme court
This integration will make playlists more dynamic and responsive to the user’s emotional needs, beyond basic moods. For instance, a playlist for studying might incorporate songs with low energy and calming lyrics based on a user’s stated preference.
Impact of Artificial Intelligence on the Algorithm
Artificial intelligence (AI) will be crucial in driving the next generation of Spotify’s playlist algorithms. AI-powered machine learning models will analyze massive datasets of user listening history, preferences, and feedback to identify patterns and predict future listening choices. This allows for more accurate predictions of what users will enjoy, leading to higher satisfaction rates and more engaging experiences.
Ever wondered how Spotify’s algorithm crafts those perfect playlists? It’s fascinating how it learns your tastes. Speaking of fascinating, did you hear about the Steelers hiring Arthur Smith as their new offensive coordinator? arthur smith hired steelers offensive coordinator That’s a major move, and perhaps a sign of the team’s renewed focus on the field.
Either way, it reminds me how complex and intriguing the algorithms behind these seemingly simple playlists are. Back to those Spotify playlists – they’re truly a marvel of modern technology!
Examples include predicting a user’s interest in a new artist based on similar listening patterns or recommending a specific genre based on a user’s current emotional state.
Examples of Algorithm Evolution to Meet Future User Needs
Algorithms will evolve to anticipate user needs, adapting to different listening contexts. For instance, a user might specify a need for background music while working, resulting in the algorithm providing a playlist of instrumental pieces, classical music, or ambient sounds. Additionally, playlists designed for specific activities, such as studying, exercising, or relaxing, will become more sophisticated, incorporating elements like tempo, rhythm, and emotional content to maximize user engagement.
Potential Improvements or Features for the Algorithm
- Integration of user mood data for personalized playlists: This will allow the algorithm to create playlists tailored to specific emotional states. For example, a playlist designed for relaxation might include calming instrumental music, while a playlist for energy might incorporate upbeat tracks. This feature could be implemented by incorporating data from user-input mood indicators or by analyzing user listening history for patterns associated with specific moods.
- Integration of social listening data: The algorithm could incorporate social listening data, such as trending topics on social media platforms related to music, to identify emerging artists or genres and adapt playlists accordingly. This feature can be implemented by monitoring social media trends and incorporating relevant information into the algorithm’s analysis.
- Dynamic playlist adaptation based on real-time listening behavior: The algorithm could adjust playlists in real-time based on the user’s listening behavior. If a user skips several songs, the algorithm could remove those songs from the playlist. If a user repeatedly listens to a specific song, the algorithm could add more similar songs. This dynamic adaptation could lead to playlists that are more tailored to the user’s current preferences and listening experience.
Comparison with Other Platforms
Spotify’s dominance in the music streaming landscape is undeniable, but its approach to playlist algorithms isn’t without competitors. Understanding how other platforms function reveals both strengths and weaknesses in the ever-evolving world of algorithmic music curation. Different services prioritize distinct user experiences, resulting in a variety of approaches to recommending music.The music streaming industry is a dynamic ecosystem, with various services vying for user attention.
Comparing Spotify’s algorithms to those of its competitors provides a broader perspective on the diverse strategies employed in the sector and highlights the factors that contribute to each platform’s success.
Algorithm Type
Different streaming services employ varying approaches to their algorithms. Spotify, for example, uses a sophisticated collaborative filtering system that considers user listening history, preferences, and social interactions. This allows for highly personalized recommendations. In contrast, Apple Music’s algorithm leans towards a blend of collaborative filtering and content-based filtering, focusing on genre and artist relationships. Other platforms, like YouTube Music, rely more heavily on content-based filtering, recommending similar artists and songs based on a user’s existing library.
User Interface
The user interface significantly influences how users interact with the algorithm’s recommendations. Spotify’s interface often provides clear visual cues about the algorithm’s logic, such as prominently displaying personalized recommendations and playlists. Apple Music, on the other hand, may integrate its recommendations more seamlessly into the overall music discovery experience. This integration might lead to less explicit feedback on how the algorithm functions.
Playlist Features
The customization and creation of playlists are crucial aspects of music streaming. Spotify allows for extensive playlist creation, offering advanced features such as collaborative playlists and the ability to follow artists and other users’ playlists. Apple Music also provides strong playlist creation tools, but its focus might be slightly less on community-driven playlist building. Other services may focus on curated playlists or specific genre recommendations, providing a different user experience.
Comparison Table
Feature | Spotify | Apple Music | Other Platform (e.g., YouTube Music) |
---|---|---|---|
Algorithm Type | Collaborative filtering, incorporating social interactions | Blend of collaborative and content-based filtering | Predominantly content-based filtering |
User Interface | Clear visualization of personalized recommendations | Seamless integration of recommendations into overall experience | Focus on discovery within a broader platform |
Playlist Features | Extensive playlist creation tools, collaborative playlists, user following | Strong playlist creation, potentially less emphasis on community-driven playlists | Curated playlists, genre-specific recommendations |
Conclusive Thoughts
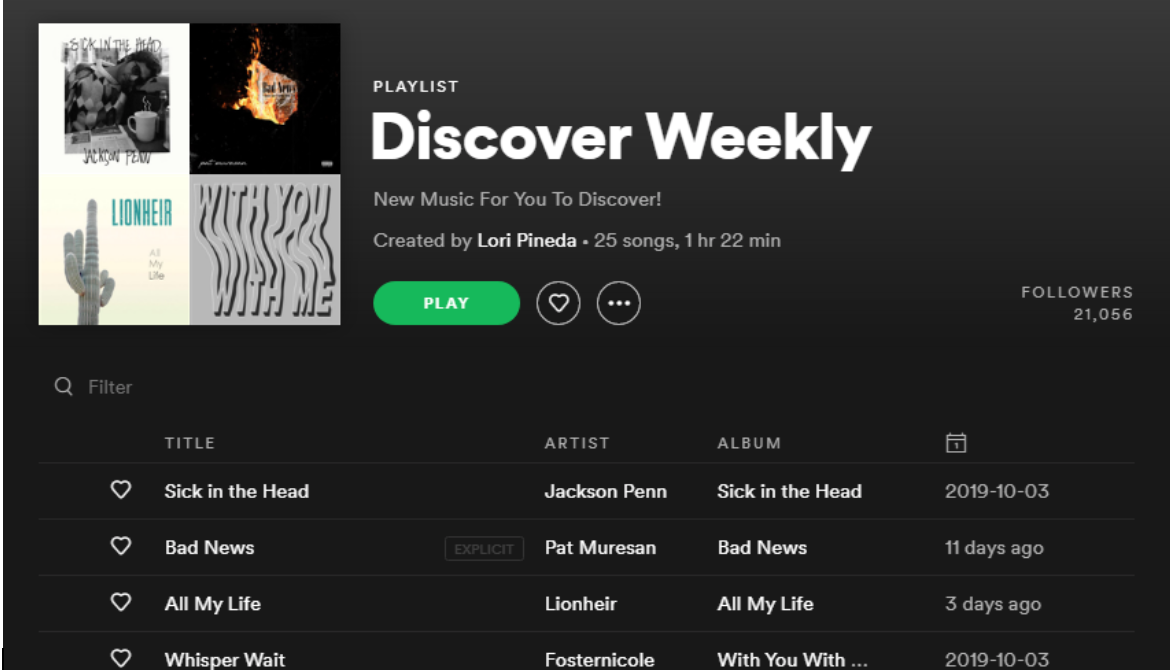
In conclusion, the lista reproduccion spotify algoritmo is a complex and powerful system that has a profound impact on user behavior, music discovery, and the music industry as a whole. While these algorithms bring us a wealth of music and tailored experiences, it’s important to acknowledge potential biases and limitations. As technology evolves, so too will these algorithms, continuing to shape our musical future.
Questions and Answers
What are the different types of Spotify playlist algorithms?
Spotify utilizes a combination of collaborative and personalized algorithms. Collaborative algorithms consider the listening habits of users with similar tastes, while personalized algorithms focus on individual user preferences.
How do Spotify playlists impact music discovery?
Playlists act as curated gateways to new music. Users frequently discover new artists and genres through the recommendations provided by these algorithms.
Can users influence Spotify’s algorithm recommendations?
Users can influence recommendations by actively creating and curating playlists, providing feedback, and utilizing the platform’s features to personalize their listening experience.
What is the impact of Spotify’s algorithms on artists?
Spotify’s algorithms can significantly impact an artist’s visibility and popularity, providing opportunities for wider exposure and potential commercial success.