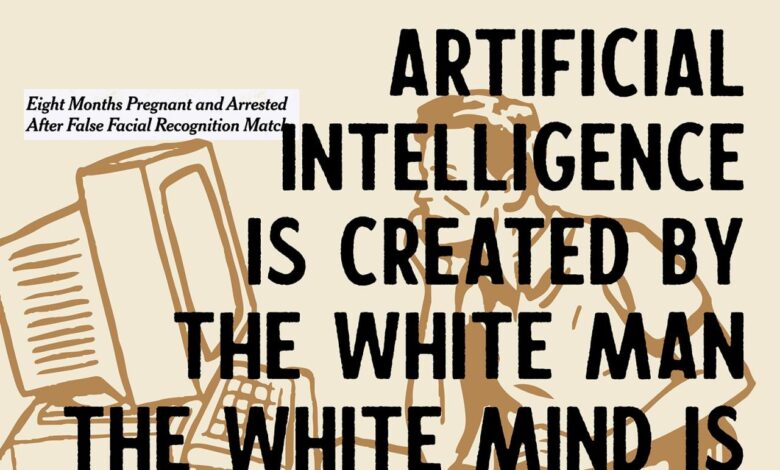
AI Bias Racism and Harassment
Inteligencia artificial acoso racismo is a complex issue with far-reaching consequences. AI systems, while powerful tools, can reflect and amplify societal biases, leading to harassment and discrimination, particularly against racial minorities. This article explores the mechanisms behind this bias, examining its impact on vulnerable communities, and proposes potential solutions.
From loan applications to hiring processes, AI systems are increasingly used in crucial decision-making areas. When these systems are trained on biased data, they can perpetuate harmful stereotypes and inequalities. This article delves into the historical roots of these biases, highlighting the critical need for ethical development and responsible deployment of AI.
Defining Artificial Intelligence Bias
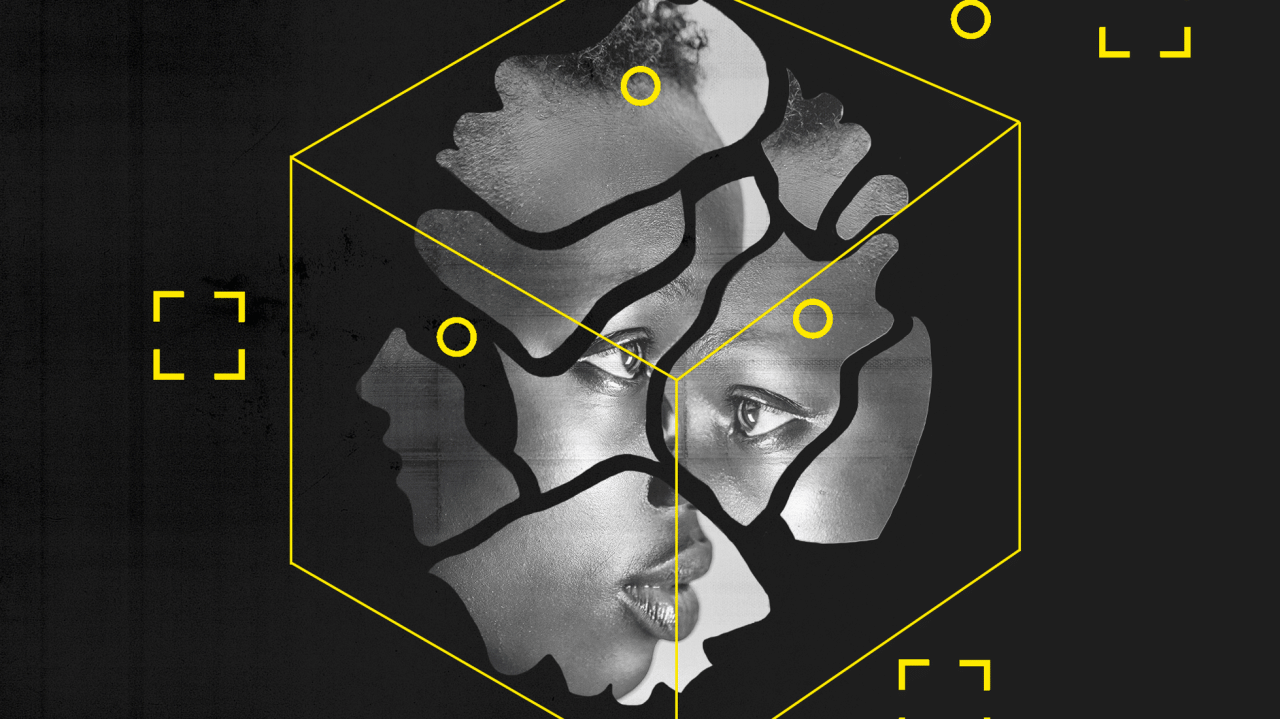
Artificial intelligence systems, while powerful, are not immune to the biases present in the data they are trained on. These biases can manifest in various forms, leading to unfair or discriminatory outcomes. Understanding these biases is crucial for developing responsible and equitable AI applications. Recognizing the sources of bias and the mechanisms through which they are perpetuated is essential for mitigating their impact.AI bias is a critical issue in the development and deployment of AI systems.
It arises from various sources, from the data used to train the models to the algorithms themselves and even the humans who design and implement them. These biases can have significant consequences, leading to unfair or discriminatory outcomes in areas like loan applications, hiring processes, and even criminal justice. Addressing these biases is essential to ensure fairness and equity in AI applications.
Types of AI Bias, Inteligencia artificial acoso racismo
AI bias encompasses several forms. Understanding these diverse forms helps us recognize and address the potential for harm. Data bias, algorithmic bias, and human bias all contribute to the problem.
Data Bias
Data bias occurs when the training data reflects existing societal biases. For example, if a facial recognition system is trained primarily on images of light-skinned individuals, it may perform poorly on images of darker-skinned individuals. This reflects the historical and ongoing underrepresentation of diverse groups in data sets. Biased data can lead to inaccurate or unfair outcomes, as the AI system will learn and perpetuate these inaccuracies.
Algorithmic Bias
Algorithmic bias refers to the inherent biases embedded within the algorithms used to train AI systems. These biases can arise from the mathematical formulations themselves or from how the algorithms are designed and implemented. A simple example is a loan application algorithm that disproportionately denies loans to applicants from certain racial groups. These algorithms can perpetuate existing inequalities, even if the developers don’t explicitly intend for that outcome.
Human Bias
Human bias in AI development and deployment refers to the biases that human developers and users introduce into the system. This can include conscious or unconscious biases in the design process, data collection, or the interpretation of AI outputs. A developer’s preconceived notions or societal stereotypes can inadvertently be encoded into the AI system. For instance, a hiring algorithm designed by a team predominantly composed of people with a certain background might inadvertently favor candidates with similar backgrounds.
Mechanisms of Bias Perpetuation
AI systems can perpetuate existing societal biases through several mechanisms. These mechanisms include the reinforcement of stereotypes through repeated exposure, amplification of existing disparities, and the creation of new forms of discrimination. For example, if an AI system used in loan applications consistently denies loans to applicants from certain racial or ethnic groups, this reinforces the idea that these groups are less creditworthy.
This reinforcement leads to a perpetuation of existing societal biases and can exacerbate inequalities.
Comparison of AI Bias Types
Bias Type | Description | Example | Mechanism |
---|---|---|---|
Data Bias | Bias present in the data used to train the AI system. | Facial recognition system trained on predominantly light-skinned faces. | Reflects existing societal underrepresentation. |
Algorithmic Bias | Bias embedded in the algorithms themselves. | Loan application algorithm disproportionately denying loans to certain ethnic groups. | Mathematical formulations or design flaws. |
Human Bias | Bias introduced by human developers and users. | Hiring algorithm designed by a team favoring candidates with similar backgrounds. | Conscious or unconscious biases in the design, data collection, or interpretation. |
Examining AI’s Role in Harassment and Discrimination
Artificial intelligence (AI) systems, while offering numerous benefits, can inadvertently perpetuate and amplify existing societal biases, including those related to race and ethnicity. This is particularly concerning in the context of harassment and discrimination, where AI tools can be misused to target vulnerable groups, often with devastating consequences. The potential for AI to exacerbate existing inequalities necessitates careful examination of its implementation and potential biases.AI systems trained on biased data can learn and reproduce those biases, leading to discriminatory outcomes.
This can manifest in various forms, from algorithmic decision-making in loan applications to facial recognition systems misidentifying individuals from minority groups. Understanding how these biases emerge and how they can be mitigated is crucial to prevent AI from becoming a tool for further marginalization.
Examples of AI-Perpetuated Racial Stereotypes
AI systems trained on historical data often reflect and amplify existing societal biases, particularly racial stereotypes. For instance, facial recognition software has demonstrated a higher error rate in identifying individuals with darker skin tones, leading to misidentification and potential misjudgment. Similarly, AI systems used in criminal justice may perpetuate racial bias in sentencing or parole decisions if trained on data reflecting historical biases.
This highlights the importance of ensuring that AI training data is representative and free from bias.
Case Studies of AI-Driven Racial Discrimination
Several case studies illustrate the potential for AI to perpetuate racial discrimination. One example involves a loan application system that disproportionately denied loans to applicants from minority communities. This system, trained on historical data reflecting existing racial disparities, further exacerbated economic inequalities. Another case study shows how AI-powered recruitment tools can unintentionally discriminate against candidates from underrepresented racial groups, leading to a lack of diversity in the workforce.
These examples demonstrate the real-world consequences of AI bias and underscore the need for careful scrutiny and responsible development of AI systems.
Potential for AI to Exacerbate Societal Inequalities
AI systems have the potential to exacerbate existing societal inequalities by reinforcing existing stereotypes and biases. For example, if AI systems are used in the hiring process and are trained on data reflecting historical hiring patterns, they might perpetuate existing biases and limit opportunities for individuals from underrepresented groups. Similarly, in the criminal justice system, AI systems used for risk assessment could unfairly target minority communities, leading to harsher sentences and increased incarceration rates.
The potential for AI to amplify existing societal inequalities demands careful consideration and proactive measures to mitigate bias.
The potential for AI to perpetuate biases like racism and harassment is a serious concern. It’s crucial to examine how algorithms are trained and how they can be used responsibly. Interestingly, the career trajectory of a public figure like Chita Rivera, as highlighted in chita rivera key moments career , shows how individuals can overcome obstacles and achieve success.
Ultimately, we need to critically assess the impact of AI on society, particularly when considering its potential to amplify existing prejudices.
Different Ways AI Can Be Employed in Harmful Activities
Type of Harmful Activity | Specific AI Application | Potential Impact |
---|---|---|
Racial Profiling | Facial recognition systems trained on biased data | Misidentification and targeting of individuals from minority groups |
Discriminatory Lending Practices | AI-powered loan application systems trained on biased data | Increased financial disparities and marginalization of minority communities |
Biased Recruitment Tools | AI-powered recruitment tools trained on biased data | Lack of diversity in the workforce and limited opportunities for underrepresented groups |
Reinforcement of Existing Racial Stereotypes | AI systems used in media, entertainment, or social networks trained on biased data | Perpetuation of stereotypes and negative perceptions of minority groups |
Analyzing the Impact on Vulnerable Communities
AI systems, while offering potential benefits, can perpetuate and amplify existing societal biases, disproportionately impacting vulnerable communities. This amplification can manifest in various forms of harassment and discrimination, leading to severe and long-lasting consequences. Understanding these impacts is crucial for developing ethical guidelines and mitigating harm.The amplification of existing biases in AI systems can have devastating consequences for vulnerable communities.
This is not just a theoretical concern; it’s a real-world issue with tangible impacts on individuals’ lives, opportunities, and well-being. Recognizing these patterns and working to address them is vital for creating a more just and equitable future.
Specific Vulnerable Communities
Various communities face heightened vulnerability to AI-driven harassment and discrimination. These groups often experience systemic disadvantage and marginalization, making them particularly susceptible to the negative impacts of biased AI.
- Racial minorities:
- Women:
- LGBTQ+ individuals:
- People with disabilities:
- Low-income individuals:
Pre-existing racial biases in datasets can lead to discriminatory outcomes in loan applications, hiring processes, and even criminal justice. For example, an AI system trained on biased data might unfairly deny loans to individuals from a particular racial background, perpetuating existing economic disparities.
Gender bias in AI can lead to unfair treatment in areas like hiring, promotion, and even healthcare. AI systems trained on data reflecting historical gender biases can perpetuate these biases, resulting in unfair outcomes for women.
AI systems trained on data containing stereotypes about LGBTQ+ identities may exhibit biases in areas like housing, employment, and access to healthcare. This can lead to discriminatory outcomes, including denial of services or targeted harassment.
AI systems that rely on specific forms of data collection or analysis may fail to accurately capture the needs and experiences of people with disabilities. This can lead to exclusion from services or the creation of systems that are inaccessible.
AI systems trained on data reflecting socioeconomic disparities may perpetuate these inequalities. For example, AI systems used in loan applications might disproportionately deny loans to individuals from low-income backgrounds.
Negative Impacts on Vulnerable Communities
AI systems can negatively impact vulnerable communities in various ways, exacerbating existing inequalities and creating new forms of discrimination.
- Reinforcement of stereotypes:
- Limited access to opportunities:
- Increased harassment and discrimination:
- Erosion of trust and social cohesion:
AI systems trained on biased data often reinforce existing stereotypes about specific communities. This can lead to the perpetuation of negative societal perceptions and further marginalization of these groups.
AI systems used in decision-making processes, such as loan applications or hiring, can discriminate against members of vulnerable communities, limiting their access to opportunities and resources.
AI-powered tools used for surveillance or monitoring can be used to target members of vulnerable communities, leading to increased harassment and discrimination.
The perceived unfairness and bias of AI systems can erode trust in institutions and contribute to social unrest.
Long-Term Consequences of AI Bias
The long-term consequences of AI bias on vulnerable communities can be significant and far-reaching. These consequences include the perpetuation of existing inequalities, the creation of new forms of discrimination, and the erosion of trust in institutions.
- Increased socioeconomic disparities:
- Perpetuation of systemic disadvantage:
- Reduced social mobility:
AI bias can exacerbate existing socioeconomic disparities by limiting access to opportunities and resources for vulnerable communities.
AI bias can help perpetuate systemic disadvantage, limiting the ability of these communities to progress and thrive.
AI bias, specifically in areas like harassment and racism, is a serious concern. It’s important to understand how algorithms can perpetuate existing societal prejudices. Recent news about stars like Harley, Johnston, Oettinger, and Benn, caught my eye and made me think about the broader impact of these issues. Ultimately, responsible AI development must prioritize fairness and avoid amplifying existing societal inequalities.
AI bias can reduce social mobility by creating barriers to education, employment, and other opportunities.
Ethical Implications for Vulnerable Groups
The ethical implications of AI systems for vulnerable communities are profound and require careful consideration. These systems should be designed and implemented with the well-being of these communities in mind.
- Transparency and accountability:
- Fairness and equity:
- Community engagement:
AI systems should be transparent and accountable for their decisions, especially when impacting vulnerable communities.
AI systems should be designed to promote fairness and equity, minimizing bias and ensuring that all communities benefit from their use.
Vulnerable communities should be involved in the design and implementation of AI systems to ensure their needs and concerns are considered.
Vulnerability Levels Across Communities
Community | Level of Vulnerability | Explanation |
---|---|---|
Racial Minorities | High | Historical and systemic discrimination, potential for biased data sets. |
Women | Medium-High | Gender bias in data sets, unequal representation in leadership positions. |
LGBTQ+ Individuals | Medium-High | Lack of representation in data sets, potential for discriminatory algorithms. |
People with Disabilities | Medium | Lack of accessibility features in AI systems, potential for exclusion from services. |
Low-Income Individuals | Medium-High | Bias in data sets reflecting socioeconomic disparities, potential for unfair access to resources. |
Exploring Mitigation Strategies and Solutions
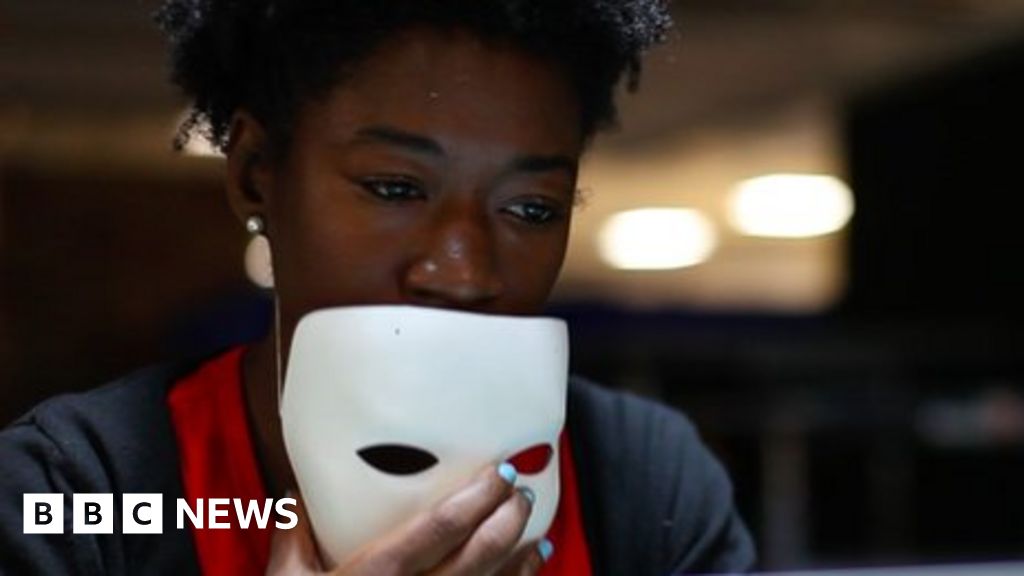
AI systems, while powerful tools, can unfortunately perpetuate existing societal biases, leading to harmful outcomes like harassment and discrimination. Addressing these biases requires a multifaceted approach that considers the entire AI lifecycle, from data collection and algorithm design to deployment and evaluation. Effective mitigation strategies demand collaboration between policymakers, developers, and researchers to ensure fairness and accountability.Addressing AI bias in the context of harassment and racism necessitates a proactive and comprehensive strategy.
This involves understanding the root causes of bias within the data and algorithms, implementing techniques to detect and mitigate harmful outcomes, and promoting diverse perspectives in the development process. Furthermore, transparent guidelines and accountability mechanisms are crucial to prevent the misuse of AI systems.
Potential Strategies for Mitigating AI Bias
Strategies for mitigating AI bias are crucial to prevent harmful outcomes. These strategies involve careful consideration of data sources, algorithm design, and ongoing monitoring. Bias can stem from various sources, including the representation of different groups in the data, the inherent assumptions encoded within the algorithms, or the lack of diversity in the development team.
- Data Preprocessing and Augmentation: Identifying and addressing biases in training data is paramount. This can involve techniques such as data augmentation, where existing data is modified to better represent underrepresented groups. Data cleaning and removal of irrelevant or biased attributes are also important. For instance, if an algorithm is trained on data that disproportionately portrays a particular gender in a negative light, the training data can be augmented with more balanced representations to avoid perpetuating harmful stereotypes.
- Algorithmic Fairness and Transparency: Developing algorithms that explicitly consider fairness criteria is essential. This involves implementing fairness metrics to assess the impact of algorithms on different groups. Furthermore, methods for making AI systems more transparent and understandable are needed to help identify and rectify biases. For example, algorithms designed to assess loan applications can be scrutinized for gender bias by analyzing the impact on approval rates of each gender group.
- Diverse Teams and Inclusion: AI development teams should reflect the diversity of the populations they aim to serve. This includes incorporating individuals from various backgrounds, perspectives, and experiences. This diverse perspective can help identify potential biases and develop solutions to mitigate them. For example, a team designing an AI system for medical diagnosis should include doctors, patients, and researchers from diverse backgrounds to avoid overlooking cultural or demographic factors that may affect the results.
Improving Fairness and Equity in AI Systems
Implementing fairness and equity in AI systems requires a multi-pronged approach. This includes not only the technical aspects but also the social and ethical considerations. The ultimate goal is to create systems that treat all groups fairly and equitably.
- Auditing and Monitoring: Regular audits of AI systems can identify and address bias in real-time. Continuous monitoring of the system’s performance across different demographic groups can highlight potential issues and allow for corrective actions. For instance, a company using AI to assess job applicants should monitor the system’s performance for gender bias by tracking the acceptance rates of male and female applicants.
- Establishing Accountability Mechanisms: Creating clear accountability mechanisms for AI systems is crucial. This involves establishing clear lines of responsibility for addressing biases and ensuring that developers and organizations are held accountable for the impact of their systems. For example, a social media platform using AI to moderate content should have a clear process for addressing complaints about bias in its moderation policies.
The Role of Policymakers and Developers
Policymakers and developers play critical roles in shaping the future of AI and addressing its potential biases. Clear guidelines and regulations are necessary to ensure responsible AI development and deployment.
- Developing Ethical Guidelines: Policymakers should create clear ethical guidelines for the development and deployment of AI systems. These guidelines should address issues of bias, fairness, and accountability. For example, government agencies could create guidelines for companies that use AI in loan applications or criminal justice systems to ensure fairness and transparency.
- Promoting Research and Education: Both policymakers and developers should support research and education initiatives to advance our understanding of AI bias and develop strategies to mitigate it. For instance, funding research into algorithmic fairness and bias detection can help to advance the field and provide effective solutions.
A Table of Approaches to Fairness and Accountability in AI Systems
Approach | Description | Example |
---|---|---|
Data Auditing | Identifying and correcting biases in training data. | Removing data points exhibiting racial bias in loan application datasets. |
Algorithmic Fairness | Designing algorithms that explicitly consider fairness criteria. | Developing algorithms for loan applications that do not discriminate against certain demographic groups. |
Transparency | Making AI systems understandable and explainable. | Providing explanations for AI-driven decisions in medical diagnosis systems. |
Accountability | Establishing clear lines of responsibility for AI systems. | Creating a mechanism for users to file complaints about AI-driven decisions. |
Analyzing the Historical Context
Artificial intelligence systems, trained on vast datasets, inherit and amplify existing societal biases. Understanding the historical roots of these biases is crucial to developing fairer and more equitable AI. This analysis delves into the historical factors that have shaped the data used to train AI systems, highlighting how historical injustices are encoded within these datasets.The biases embedded in AI systems are not simply a modern problem; they reflect and perpetuate historical patterns of inequality.
From the representation of marginalized groups in datasets to the very algorithms used, the echoes of past discrimination reverberate in the outcomes of today’s AI applications. This historical context reveals a crucial link between the past and the present, emphasizing the need for proactive measures to mitigate bias in AI development.
The disturbing issue of AI-driven harassment and racism is a real concern, and it’s important to address it head-on. Looking at the dazzling array of red carpet photos from the Critics’ Choice Awards critics choice awards red carpet photos reminds us of the beauty and diversity in the world, a stark contrast to the harmful algorithms that perpetuate these biases.
Ultimately, we need to find ways to use AI responsibly and combat these harmful trends.
Historical Factors Contributing to Bias in AI Data
Historical datasets often lack diversity, reflecting the limited representation of marginalized groups in past societies. This underrepresentation translates directly into the data used to train AI systems, resulting in biased outcomes. For example, historical crime records, frequently used to train predictive policing algorithms, often disproportionately target minority communities, reflecting and reinforcing existing societal prejudices.
Reflection of Societal Biases in AI Training Datasets
Societal biases have been consistently reflected in the datasets used for AI training. These biases manifest in various forms, including gender bias, racial bias, and socioeconomic bias. For instance, if a dataset used to train an image recognition system predominantly features images of light-skinned individuals, the system may struggle to recognize or categorize individuals with darker skin tones.
Historical Context of Racism and Discrimination Shaping AI Biases
Historical racism and discrimination have profoundly shaped the data used to train AI systems. Datasets often perpetuate harmful stereotypes and prejudices, leading to discriminatory outcomes in areas like loan applications, hiring processes, and criminal justice. The legacy of discriminatory practices, such as redlining in housing, can be found embedded in datasets used to train AI systems for real estate appraisal or risk assessment.
AI’s role in perpetuating racism and harassment is a serious concern. Recent events, like the complex dynamics surrounding the trump voters iowa caucus , highlight how existing societal biases can be amplified by algorithms. Understanding how these technologies can exacerbate existing prejudices is crucial to developing responsible AI applications and ensuring fairness in society.
Encoding of Historical Injustices in AI Development Data
Historical injustices are often encoded within the data used for AI development. This includes data reflecting past discriminatory practices, biased social norms, and systemic inequalities. For example, historical records of incarceration rates, often disproportionately affecting certain racial or ethnic groups, can be incorporated into datasets used to predict recidivism, potentially perpetuating existing biases.
Timeline of Key Historical Events Related to Bias in Data and AI Systems
- 1950s-1960s: Development of early AI systems, often trained on limited and biased datasets reflecting the societal norms of the time.
- 1970s-1980s: Increased use of statistical models and algorithms, which often inherited and amplified existing biases in the underlying data.
- 1990s: The rise of the internet and the digitalization of data, leading to the accumulation of large datasets that reflected existing societal biases, further exacerbating the issue.
- 2000s-present: The explosive growth of AI and machine learning, combined with the increasing availability of vast datasets, has highlighted the profound impact of historical biases on AI systems. Instances of biased outcomes in areas like loan applications and criminal justice have become increasingly apparent.
Illustrating the Problem Through Examples: Inteligencia Artificial Acoso Racismo
AI systems, while powerful tools, are not immune to the biases present in the data they are trained on. These biases, often reflecting societal prejudices, can be amplified and perpetuated by AI, leading to discriminatory outcomes in various real-world applications. Understanding these examples is crucial to developing mitigation strategies and ensuring fairness and equity in AI deployments.
Loan Applications
Loan applications frequently utilize AI to assess creditworthiness. However, if the training data predominantly reflects the financial history of a particular demographic group, the AI may unfairly favor applicants from that group, potentially excluding others. This can lead to systemic inequities in access to credit, hindering economic opportunities for underrepresented populations. For instance, historical lending patterns have disproportionately denied loans to minority borrowers, and AI models trained on this biased data may perpetuate this pattern.
Hiring Processes
AI-powered applicant screening systems are increasingly used in hiring processes. If these systems are trained on historical hiring data that reflects existing biases against certain demographic groups or skill sets, they may inadvertently screen out qualified candidates from these groups. This can lead to a lack of diversity in the workforce and limit opportunities for individuals from underrepresented backgrounds.
For example, a system trained on data from a predominantly male workforce might unconsciously favor male candidates over equally qualified female applicants.
Criminal Justice
AI systems are being employed in criminal justice to predict recidivism and assess risk. However, if the data used to train these systems reflects existing racial biases in the criminal justice system, the AI may unfairly label individuals from certain racial groups as higher risk, leading to harsher sentencing or increased surveillance. This can exacerbate existing inequalities and create a self-fulfilling prophecy, where individuals from marginalized groups are disproportionately targeted.
For instance, if a system is trained on data indicating a higher rate of recidivism among a specific racial group, it might incorrectly predict higher recidivism rates for individuals from that group, even if they do not exhibit higher risk.
AI bias, like racism and harassment, is a serious concern. It’s a global issue, and unfortunately, it’s not just limited to algorithms. The recent Taiwan election, particularly the China Lai Ching Te campaign, taiwan election china lai ching te , highlights how political narratives can amplify existing prejudices. Ultimately, these issues demonstrate the need for ongoing scrutiny and ethical considerations in AI development.
Automated Discriminatory Practices
AI can automate discriminatory practices in various domains. For example, an AI-powered system designed to identify and flag suspicious activity in financial transactions might unfairly target individuals from certain ethnic backgrounds or communities, leading to unwarranted scrutiny and financial hardship. Such an instance could arise from training data that reflects existing societal prejudices or stereotypes.
Table: Examples of AI Bias in Different Applications
Application | Type of Bias | Example |
---|---|---|
Loan Applications | Demographic bias | AI system consistently denies loans to applicants from a specific racial or ethnic group, despite comparable financial profiles. |
Hiring Processes | Gender bias | AI-powered screening tools systematically favor male candidates over equally qualified female candidates. |
Criminal Justice | Racial bias | AI-powered recidivism prediction tools label individuals from a particular racial group as higher risk, leading to harsher sentencing or increased surveillance. |
Financial Transactions | Ethnic bias | AI system unfairly flags transactions of individuals from a specific ethnic background as suspicious, leading to unwarranted scrutiny. |
Proposing Ethical Guidelines and Frameworks
AI systems are increasingly woven into the fabric of our lives, impacting everything from healthcare to finance. However, the potential for bias in these systems presents a significant ethical challenge. Developing and deploying AI responsibly requires a proactive approach to mitigating bias and promoting fairness. Robust ethical guidelines and frameworks are essential to ensure these powerful tools are used for the benefit of all.To effectively address the issue of bias in AI, we need a multi-faceted approach that combines clear guidelines with practical assessment tools.
This involves not only understanding the sources of bias but also creating systems that actively work against its manifestation.
Ethical Guidelines for AI Development and Deployment
Establishing clear ethical guidelines is crucial for responsible AI development. These guidelines should be comprehensive, covering various stages of the AI lifecycle, from data collection to deployment and ongoing monitoring. A robust framework needs to encompass the entire process to prevent bias from creeping in at any point.
- Data Collection and Preprocessing: Data used to train AI models should be diverse and representative of the population it will serve. Bias in training data directly translates into bias in the resulting AI. This requires careful consideration of the sources and characteristics of the data used, proactively identifying and mitigating potential biases embedded within the datasets. Techniques like data augmentation and debiasing methods can be used to address biases during data preprocessing.
- Model Design and Evaluation: AI models should be designed with fairness and equity in mind. Bias detection mechanisms need to be incorporated into the model design process to identify potential biases early on. Models should be evaluated not only on their accuracy but also on their fairness and equity characteristics. This means considering the impact on different groups and ensuring equitable outcomes for all users.
- Transparency and Explainability: AI systems should be designed with transparency in mind, allowing for an understanding of how decisions are made. This transparency helps identify potential biases and allows for accountability. Explaining the decision-making process of an AI system can increase trust and allow users to understand how the system works, potentially identifying any inherent biases.
- Monitoring and Auditing: AI systems need continuous monitoring and auditing to detect and address emerging biases. Regular audits and assessments of the AI system’s performance are crucial to ensure it’s operating fairly. Monitoring should track disparities in outcomes across different demographics and identify potential drifts in the system’s performance over time.
Framework for Assessing Potential Bias in AI Systems
A systematic framework is essential to identify and quantify potential biases in AI systems. This framework should include clear metrics and criteria for assessing fairness and equity. By establishing measurable standards, we can objectively evaluate the potential impact of bias on various populations.
- Bias Identification Techniques: Various techniques can be used to identify potential biases in AI models, such as demographic parity, equal opportunity, and predictive rate parity. These techniques provide quantitative measures to assess the fairness of the model’s output. A comprehensive review of the model’s performance against these metrics helps identify areas needing improvement.
- Comparative Analysis: Comparing the performance of the AI system against a benchmark or a human decision-making process can highlight potential biases. This comparative analysis can help understand where the AI system is performing differently from expected and identify the causes of such disparities. The benchmark can be an existing system or a set of established human standards.
- Impact Assessment: Evaluating the potential impact of the AI system on vulnerable communities is crucial. This involves understanding how the AI system might disproportionately affect certain groups and considering the potential consequences of these impacts. This analysis allows for the identification of specific groups that might be at risk from the system’s output.
Mitigation Strategies for Bias in AI Development
Proactive steps can be taken to mitigate the risk of bias in AI development. Addressing bias at the source, during the training process, and through ongoing evaluation is essential.
- Diverse and Representative Data Sets: Using diverse and representative data sets for training AI models can help reduce bias. A variety of viewpoints and backgrounds in the data can help the AI learn patterns and make predictions that are more equitable.
- Bias Detection and Mitigation Techniques: Incorporating bias detection and mitigation techniques into the AI development process can help ensure that the model does not perpetuate existing biases. These techniques can identify and adjust for disparities in the data to create a fairer system.
- Iterative Evaluation and Refinement: Regularly evaluating the AI system’s performance and refining the model based on the results of this evaluation can further reduce bias. This iterative process ensures the system is constantly being adjusted to ensure fairness and equity.
End of Discussion
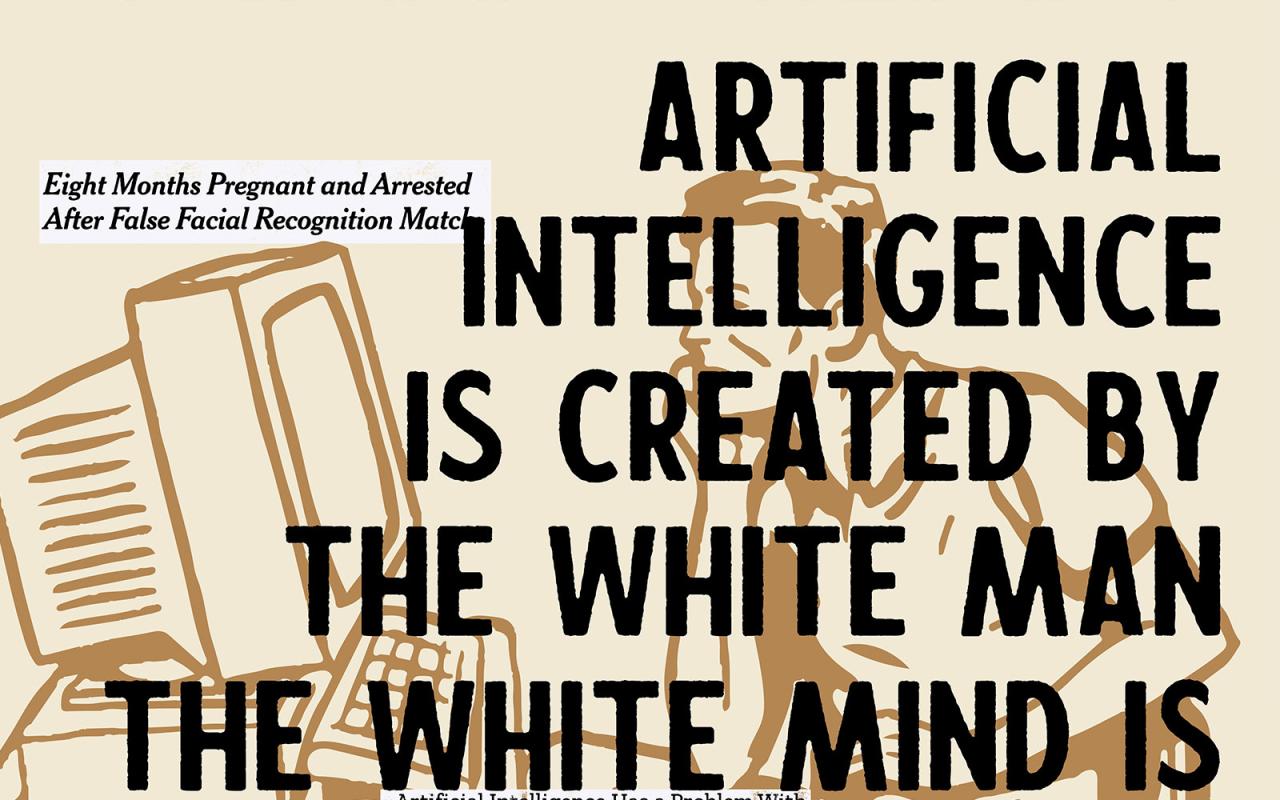
In conclusion, inteligencia artificial acoso racismo presents a significant challenge that requires careful consideration and proactive measures. Addressing bias in AI systems necessitates a multi-faceted approach, involving data analysis, algorithmic adjustments, and a commitment to ethical development. By understanding the historical context, recognizing vulnerable communities, and implementing mitigation strategies, we can strive towards a future where AI serves humanity without perpetuating harmful stereotypes and discrimination.
Key Questions Answered
How can AI systems perpetuate racial bias?
AI systems learn from data. If the training data reflects existing societal biases (like historical discrimination), the AI will likely reproduce those biases in its decision-making. This can occur through data bias, where the data itself is skewed, or algorithmic bias, where the algorithms used to process the data are designed to reinforce the bias.
What are some examples of AI-driven racial discrimination?
Examples include biased loan applications, where AI might deny loans to applicants from certain racial backgrounds. There are also instances of AI used in criminal justice, where biased algorithms might lead to harsher sentences for minority defendants.
What role do policymakers play in addressing AI bias?
Policymakers can create regulations and guidelines for the development and deployment of AI systems. This includes mandating transparency and accountability in AI algorithms, and promoting fairness and equity in their use.
How can we improve the fairness and equity of AI systems?
Improving fairness and equity involves a combination of approaches. These include careful data selection, the development of fairer algorithms, and a commitment to diverse teams in the AI development process. Regular audits and assessments of AI systems are also crucial to identify and correct bias.