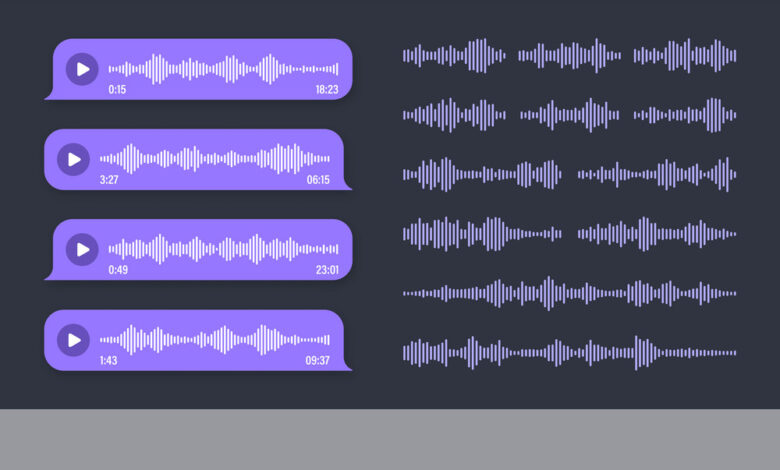
Audio Message Voice Tagging A Deep Dive
Audio mensaje voz etiqueta is revolutionizing how we interact with and understand audio data. From customer service interactions to social media analysis, the ability to accurately identify and categorize emotions, actions, and topics within spoken words opens up a wealth of possibilities. This in-depth exploration delves into the methods, applications, and future of this powerful technology.
The process involves meticulously analyzing audio recordings to extract meaningful information. This is achieved through a combination of advanced algorithms and human expertise. The accuracy of this tagging significantly impacts the quality of the insights derived from the audio data. We’ll examine various techniques and explore their effectiveness, along with their associated costs and limitations.
Defining Audio Message Voice Tagging
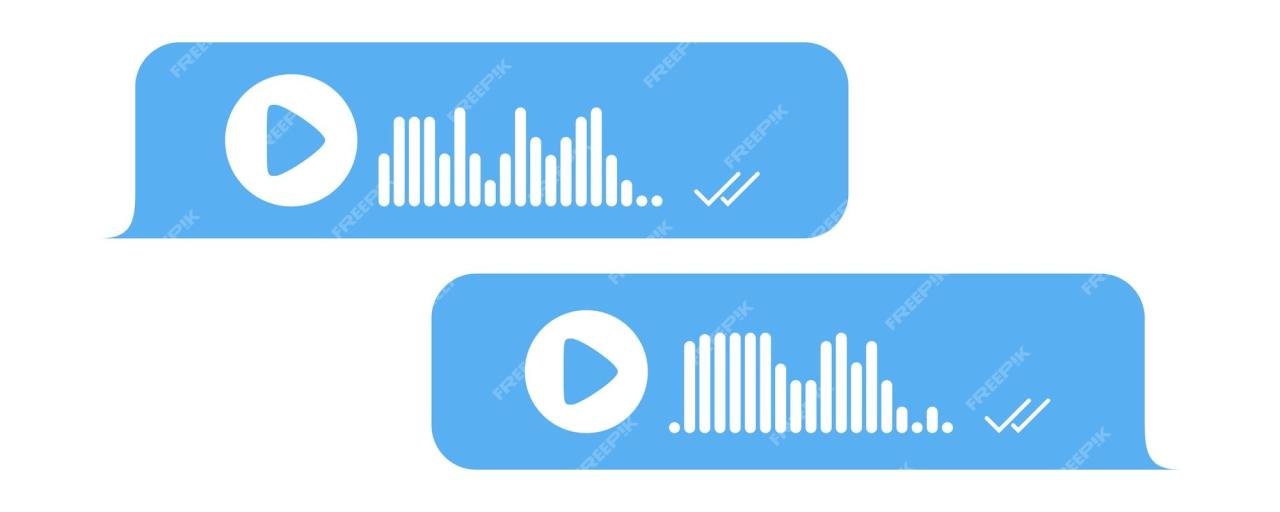
Audio message voice tagging is a powerful technique for extracting meaningful information from spoken content. It involves meticulously analyzing the vocal characteristics of an audio recording to identify and categorize specific vocal elements. This process allows for the automated extraction of key details, which can be used for a variety of applications, from sentiment analysis to content organization.
The process essentially “labels” the audio with descriptive tags based on the speaker’s tone, actions, and subject matter.
Process of Identifying and Classifying Vocal Elements
Voice tagging relies on sophisticated algorithms and machine learning models to identify and classify vocal elements. These models are trained on large datasets of audio recordings with associated labels. The process typically involves several steps: initial audio processing, feature extraction, and classification using pre-trained models. Initial processing often includes noise reduction and normalization to improve the accuracy of the analysis.
Ever wondered about audio mensaje voz etiqueta? It’s a fascinating area, but let’s be honest, sometimes the technical stuff can get a bit dry. Thinking about Taylor Swift’s recent work, particularly her “Tortured Poets Department” (check out a deep dive here ), I’ve been reflecting on how audio messaging is used in creative ways. Hopefully, these creative explorations will inspire future developments in audio mensaje voz etiqueta.
Feature extraction isolates key characteristics of the voice, such as pitch, intonation, and rhythm. Finally, the extracted features are used to classify the vocal element into predefined categories.
Types of Voice Tags
Voice tags categorize the nuances of speech into meaningful groups. These tags enable users to quickly locate specific segments within an audio message, allowing for efficient searching and retrieval. They encompass a wide spectrum of information, covering emotions, actions, and topics.
- Emotion tags represent the speaker’s emotional state. Examples include happiness, sadness, anger, and excitement. Recognizing emotional states in audio can be crucial for sentiment analysis and understanding the speaker’s intent.
- Action tags identify specific vocal actions or behaviors within the audio message. These tags include calling, singing, crying, laughing, and whispering. Such tags can be valuable for identifying specific actions or behaviors.
- Topic tags classify the subject matter of the audio message. Examples include politics, sports, music, and finance. These tags are beneficial for organizing and categorizing audio content.
Significance of Voice Tagging
Voice tagging has significant applications in various fields. It allows for efficient retrieval and organization of large audio archives, facilitates automated content analysis, and improves accessibility for individuals with disabilities. This technology empowers businesses and organizations to effectively manage and analyze vast amounts of audio data, leading to enhanced productivity and better decision-making. Examples include automated transcription and summarization of meetings, customer service interactions, and market research recordings.
Audio mensaje voz etiqueta is a fascinating field, especially when you consider how it’s used in modern communication. Thinking about how Anthony Kim’s LIV Golf Return A Detailed Look Anthony Kims LIV Golf Return A Detailed Look might be covered using this type of audio message, it’s intriguing to consider the future of sports reporting. It definitely makes me think about the power of audio messaging and how it’s evolving in various industries.
Categories of Voice Tags
The following table Artikels the categories of voice tags with example phrases.
Category | Example Phrases |
---|---|
Emotion | Happy, Sad, Angry, Excited, Scared, Frustrated, Nervous |
Action | Calling, Singing, Crying, Laughing, Whispering, Arguing, Speaking |
Topic | Politics, Sports, Music, Finance, Technology, Education, Travel |
Methods of Audio Message Voice Tagging
Audio message voice tagging is a crucial process for organizing, searching, and analyzing large volumes of audio data. Effective tagging allows for quick retrieval of specific information within recordings, making it a valuable tool in various applications, from customer service to market research. Different methods exist, each with its own strengths and weaknesses in terms of accuracy and cost.
Understanding these methods is essential for choosing the most appropriate approach for a given task.
Common Methods
Various methods are employed for audio message voice tagging, each with its own strengths and weaknesses. These methods range from relying on human expertise to leveraging advanced machine learning algorithms. A balanced approach often combines these methods to maximize accuracy and efficiency.
- Machine Learning:
- Human Annotation:
- Speech Recognition Software:
Machine learning algorithms are increasingly used for voice tagging. These algorithms are trained on large datasets of audio recordings and corresponding tags. Through patterns and correlations, the algorithm learns to identify and associate specific phrases, topics, or emotions with particular audio segments. For example, a machine learning model trained on customer service calls could identify phrases like “refund,” “problem,” or “satisfaction,” enabling automatic tagging of calls related to these topics.
I’ve been diving into audio mensaje voz etiqueta lately, exploring different ways to use voice notes for communication. It’s fascinating how quickly these messages can convey information. Recently, the tragic incident involving armorer Alec Baldwin on the set of “Rust” armorer alec baldwin rust shooting really highlighted the importance of safety protocols, not just on film sets, but in any environment where potentially dangerous tools or situations exist.
Learning about proper safety measures is definitely something that should be included in the broader context of audio mensaje voz etiqueta.
Human annotators play a vital role in voice tagging, particularly in ensuring accuracy and handling nuanced or complex speech patterns. Experienced annotators listen to audio recordings and meticulously tag them according to specific criteria. This method is particularly effective when dealing with highly specialized terminology or context-dependent expressions. For instance, annotators trained in legal terminology could accurately tag audio recordings containing complex legal jargon.
Audio message voice tagging is fascinating, especially when you consider the recent developments in the Middle East, like the Biden-Israel-Hamas cease-fire negotiations. This critical moment highlights the importance of clear communication, which audio message voice tagging could help facilitate in many different contexts. Thinking about how these voice tags could be used in various situations, like disaster relief or even just daily communication, is quite intriguing.
Speech recognition software is a crucial tool in voice tagging, converting spoken words into text. This transcribed text can then be analyzed to identify key phrases and topics. The accuracy of this method depends heavily on the quality of the audio recording and the sophistication of the speech recognition engine. For example, poor audio quality can significantly reduce the accuracy of transcription, leading to inaccurate tagging.
Comparative Analysis of Methods
A comparison of different methods reveals varying degrees of accuracy and cost.
Method | Accuracy | Cost |
---|---|---|
Machine Learning | High | Medium |
Human Annotation | High | High |
Speech Recognition | Medium | Low |
The table above illustrates the trade-offs between accuracy and cost. Machine learning offers high accuracy but comes with a medium cost associated with training and implementation. Human annotation provides high accuracy but involves significant labor costs. Speech recognition is a low-cost option but often compromises on accuracy, requiring further refinement and analysis. The optimal method will depend on specific requirements, budget constraints, and the complexity of the audio data.
Applications of Audio Message Voice Tagging
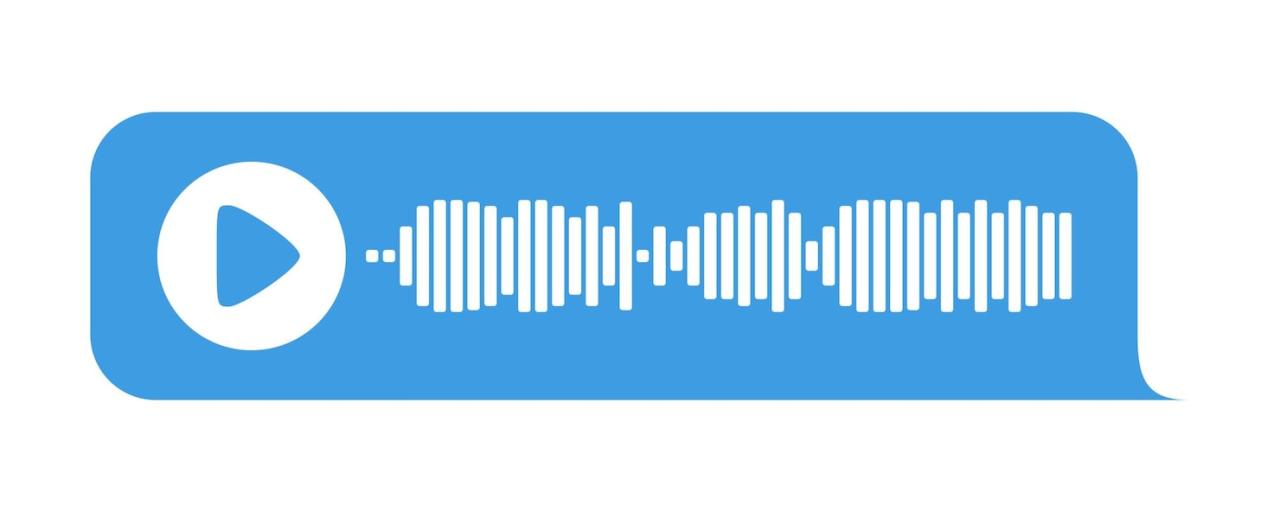
Voice tagging of audio messages is rapidly becoming a powerful tool across various industries. From customer service interactions to market research, the ability to automatically categorize and analyze spoken content offers significant advantages in efficiency, accuracy, and insight generation. This technology allows businesses to gain a deeper understanding of their customers and their needs, leading to better products, services, and overall strategies.Voice tagging goes beyond simply transcribing audio.
It unlocks the potential to understand the nuances of spoken language, including sentiment, tone, and intent. This deeper level of analysis enables businesses to extract valuable information that would otherwise remain hidden within a sea of audio data.
Customer Service
Understanding customer sentiment is crucial for effective customer service. Voice tagging enables automated analysis of customer interactions, identifying positive, negative, or neutral sentiment in real-time. This allows customer service representatives to proactively address issues and improve customer satisfaction. For example, if a large number of customers express frustration regarding a specific product feature, the company can quickly identify the problem and take corrective action, thereby reducing customer churn and maintaining loyalty.
Social Media
Analyzing public opinion and trends from audio clips on social media platforms is another significant application. Voice tagging can be used to identify recurring themes, emerging trends, and shifts in public sentiment. This capability is particularly valuable for brands and organizations that want to understand the public perception of their products, services, or initiatives. For example, during a product launch, voice tagging can quickly identify common opinions from audio comments, enabling companies to adapt their strategies in response to immediate feedback.
Market Research
Voice tagging plays a key role in market research by enabling the categorization of customer feedback based on vocal tone. This nuanced analysis reveals insights into customer preferences, concerns, and satisfaction levels, allowing researchers to gain a deeper understanding of their target market. Examples include identifying patterns in customer reactions to new product features or evaluating the impact of marketing campaigns on consumer sentiment, based on their voice tone in audio feedback.
This allows for more targeted and effective marketing strategies.
Accessibility
Voice tagging is invaluable in enhancing accessibility for individuals with hearing impairments. By automatically transcribing and tagging audio messages, individuals can access important information that might otherwise be inaccessible. This includes transcripts of meetings, customer service interactions, and educational materials. This can significantly improve the lives of individuals with hearing impairments by providing them with access to a wider range of information and communication opportunities.
Content Moderation and Analysis
Voice tagging can be applied to content moderation and analysis, particularly in social media and online forums. By identifying potentially harmful or inappropriate content, voice tagging helps maintain a safe and positive online environment. For example, identifying threats, harassment, or hate speech through voice tagging is possible, and allows moderators to take swift action.
Challenges in Audio Message Voice Tagging
Accurate voice tagging of audio messages presents numerous hurdles. While voice recognition technology has advanced significantly, it’s still not perfect. The inherent complexity of human speech, coupled with environmental factors and individual variations, creates challenges that researchers and engineers are actively addressing. The ability to precisely and reliably identify the speaker and content within an audio message remains a crucial aspect of this technology.The process of voice tagging hinges on precise identification and categorization of speech content.
I’ve been diving into audio mensaje voz etiqueta lately, and it’s fascinating how sound can convey so much emotion. Thinking about the powerful impact of spoken words reminds me of the recent piece on grief, specifically the heartbreaking story of Sloane Crosley, explored in “grief is for people sloane crosley” grief is for people sloane crosley. It made me realize how important audio messages are in expressing complex feelings, which definitely makes me appreciate the potential of audio mensaje voz etiqueta even more.
However, this accuracy is threatened by various factors, including background noise, distortions in the audio signal, and the wide spectrum of vocal variations among speakers. This is where the limitations of current voice recognition technology become apparent.
Difficulties in Accurate Voice Tagging, Audio mensaje voz etiqueta
Voice recognition systems often struggle to decipher audio signals accurately, especially when the quality is compromised. Imperfections in the audio signal, such as noise and distortions, can significantly impair the performance of voice recognition models. The system’s ability to filter out unwanted noise and interpret the underlying speech is crucial. The complexity of human speech and its inherent variations further complicate the process.
Individual vocal patterns, accents, and speaking styles all contribute to the challenges faced in accurately tagging audio messages.
Limitations of Current Voice Recognition Technologies
Current voice recognition technologies are often limited by their training data. These models are trained on a vast dataset of audio, but this dataset may not encompass the full spectrum of human speech variations, such as different accents, dialects, or speaking styles. This limitation frequently leads to errors in the tagging process. Additionally, the models may not be sufficiently robust to handle unexpected or novel speech patterns.
Handling Noisy or Distorted Audio
The presence of background noise significantly impacts the accuracy of voice tagging. This includes various forms of noise, such as ambient sounds, machinery, or even other voices in the recording. Techniques like noise reduction algorithms are employed to mitigate the negative impact of background noise on the recognition process. Similarly, distortions in the audio signal, such as echo or clipping, can severely hinder the system’s ability to accurately interpret the speech.
Advanced signal processing techniques are employed to compensate for these distortions.
Variations in Vocal Patterns and Accents
Individual vocal patterns, such as speaking rate, intonation, and rhythm, can vary significantly between speakers. Accents and dialects further add to the complexity of voice recognition, posing challenges to accurate tagging. These variations can confuse the models, leading to errors in identification and tagging. Multi-speaker models are being developed to account for these differences and improve the accuracy of voice tagging across a wider range of speakers.
Common Challenges and Potential Solutions
Challenge | Potential Solution |
---|---|
Noisy audio | Noise reduction algorithms |
Distorted audio | Advanced signal processing |
Vocal variations | Multi-speaker models |
Accents | Multilingual models |
Future Trends in Audio Message Voice Tagging
The burgeoning field of audio message voice tagging is poised for significant advancements, driven by the increasing volume of voice data and the need for efficient organization and retrieval. These advancements will reshape how we interact with and manage audio information, enabling more sophisticated applications and impacting various industries.Voice tagging is evolving beyond simple recognition, moving toward a more nuanced understanding of the spoken word, context, and even emotional tones.
This shift will unlock greater possibilities for automated analysis, summarization, and retrieval of information contained within audio messages.
Potential Advancements in Voice Tagging Technology
Voice tagging technology is expected to become more sophisticated, accurate, and efficient. This includes improvements in the speed and precision of tagging, as well as expanding the range of information that can be extracted. Improvements in algorithms and the availability of more extensive datasets will be crucial in achieving this.
Examples of Emerging Technologies
Deep learning and artificial intelligence (AI) are playing a key role in the evolution of voice tagging. Deep learning models, particularly recurrent neural networks (RNNs) and convolutional neural networks (CNNs), are adept at identifying complex patterns and relationships within audio data. AI-powered systems can analyze not only the words spoken but also the speaker’s tone, inflection, and emotional state, providing a more comprehensive understanding of the message’s content.
For example, an AI system could differentiate between a complaint and a suggestion, based on the speaker’s tone and phrasing.
Real-Time Voice Tagging
Real-time voice tagging is an exciting prospect. The ability to tag audio messages as they are being recorded would revolutionize communication management. Imagine instantly categorizing and prioritizing incoming voicemails, allowing for quicker response times and more effective handling of urgent messages. This capability would also be valuable in call centers, customer service environments, and even personal communication. The challenge lies in developing algorithms that can process and analyze audio data in real-time while maintaining high accuracy.
Integration with Other Technologies
Voice tagging technology has the potential to integrate seamlessly with other technologies, leading to even more powerful applications. Imagine a system that automatically transcribes audio messages, tags them with relevant s, and then summarizes the key points for quick access. This integration with transcription services, summarization tools, and potentially even natural language processing (NLP) will allow users to gain insights and actions from the audio message with minimal effort.
Such systems will become valuable tools for research, business analysis, and personal organization.
Timeline for Future Advancements in Voice Tagging
Predicting a precise timeline for advancements in voice tagging is difficult. However, based on the current pace of development in AI and machine learning, we can anticipate several milestones:
- Within the next 2-3 years: Improved accuracy and speed of -based tagging, particularly in well-defined domains.
- Within 5-7 years: Emergence of real-time voice tagging systems with higher accuracy, particularly in call centers and similar applications.
- Within 8-10 years: Integration of voice tagging with other technologies like transcription, summarization, and NLP will become more widespread and reliable, leading to more automated analysis and extraction of insights from audio data.
Epilogue: Audio Mensaje Voz Etiqueta
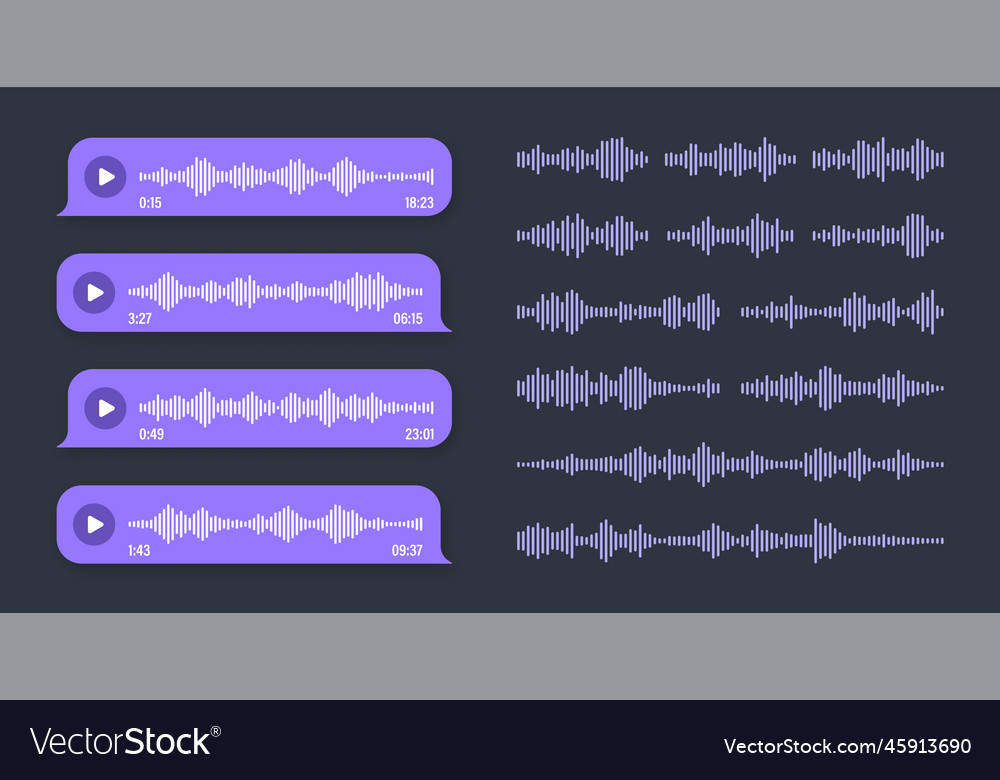
In conclusion, audio mensaje voz etiqueta is a rapidly evolving field with profound implications across numerous sectors. From improving customer service to enhancing accessibility, its potential is immense. While challenges remain, advancements in machine learning and AI promise to further refine the accuracy and efficiency of this technology. The future of audio analysis looks bright, driven by the continuous development and application of audio mensaje voz etiqueta.
Essential Questionnaire
What are some common challenges in accurate voice tagging?
Accurately tagging audio can be difficult due to factors like noisy environments, variations in accents, and speaker diversity. Distorted audio and complex vocal patterns also pose significant hurdles for accurate analysis.
How can machine learning algorithms improve the accuracy of voice tagging?
Machine learning algorithms can analyze vast amounts of audio data, identifying patterns and nuances in speech that might be missed by traditional methods. This allows for improved accuracy in identifying emotions, actions, and topics.
What is the role of human annotators in voice tagging?
Human annotators play a crucial role in training and validating machine learning models. They provide crucial feedback to ensure that the algorithms correctly categorize different aspects of speech, especially when dealing with nuances and complex vocalizations.
How does audio mensaje voz etiqueta impact accessibility?
Audio mensaje voz etiqueta can significantly improve accessibility for individuals with hearing impairments. By transcribing and tagging audio, it enables them to better understand and engage with audio content.